 |
Biwei Huang is an assistant professor at Halicioğlu Data Science Institute (HDSI) at UCSD. Her research interests include causal discovery and inference, causality-facilitated machine learning and reinforcement learning, and computational science. Her work has focused on learning and using causal world models to improve the robustness and generalization of the RL agents. Previously, she received her Ph.D. degree at CMU in 2022.
|
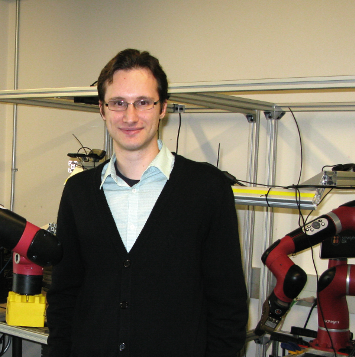 |
Sergey Levine is an Associate Professor at the University of California Berkeley and leads the Robotic AI and Learning Lab in the Berkeley AI Research Lab (BAIR). His research is focused on learning algorithms to acquire complex behaviors, especially general-purpose methods that could enable any autonomous system to learn to solve any task. His work has investigated a wide variety of RL problems, from representation learning to skill embeddings and offline RL. He received his PhD in Computer Science from Stanford University advised by Vladlen Koltun.
|
 |
Yonatan Bisk is an assistant professor of computer science at Carnegie Mellon's Language Technologies Institute. His group works on grounded and embodied natural language processing, placing perception and interaction central to language learning and understanding. His work has explored sidestepping rewards for learning agents by leveraging language grounding along with expert demonstrations. Previously, he received his PhD from the University of Illinois at Urbana-Champaign working on unsupervised Bayesian models of syntax, before spending time at USC's ISI (working on grounding), the University of Washington (for commonsense research), and Microsoft Research (for vision+language).
|
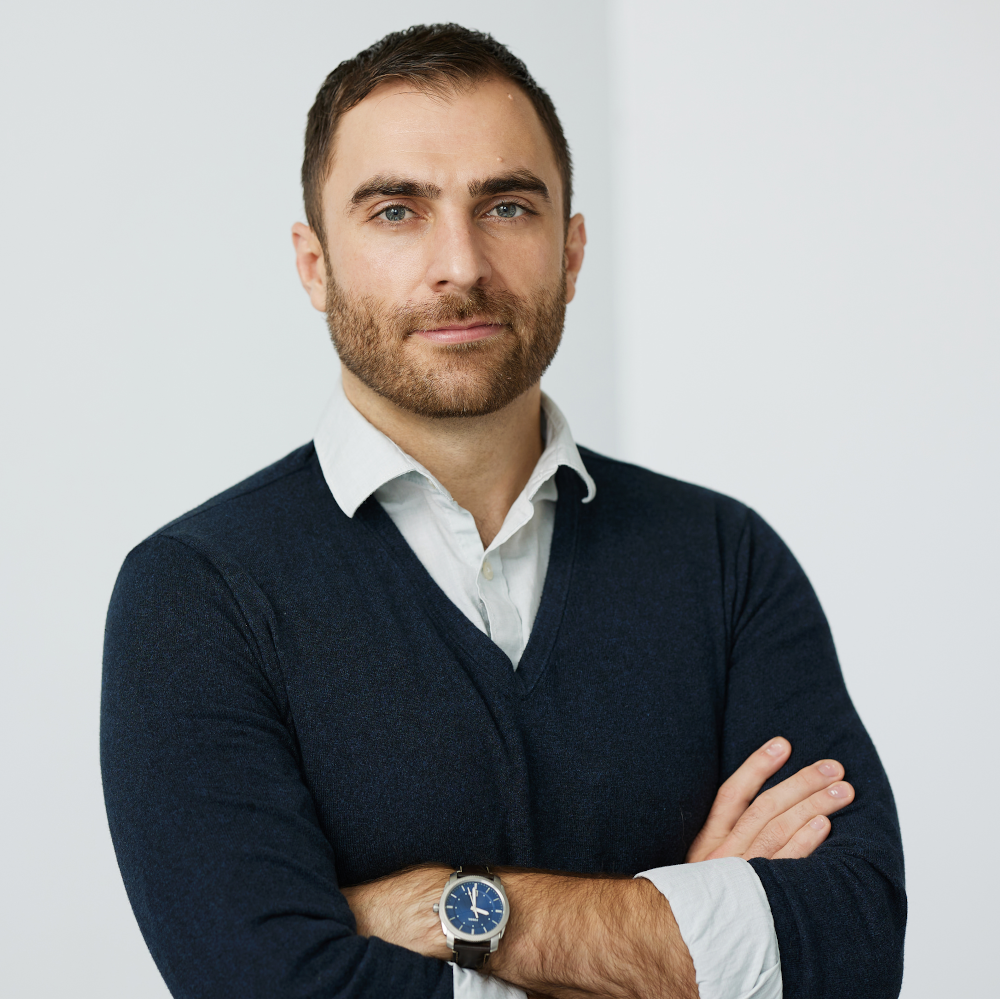 |
Glen Berseth is an assistant professor at the Université de Montréal, a core academic member of the Mila - Quebec AI Institute, Canada CIFAR AI chair, member l'Institute Courtios, and co-director of the Robotics and Embodied AI Lab (REAL). His current research focuses on machine learning and solving real-world sequential decision-making problems (planning/RL), such as robotics, scientific discovery and adaptive clean technology. The specifics of this research has covered the areas of human-robot collaboration, generalization, reinforcement learning, continual learning, meta-learning, multi-agent learning, and hierarchical learning. He also teaches courses on data science and robot learning at Université de Montréal and Mila, covering the most recent research on machine learning techniques for creating generalist agents.
|
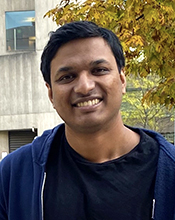 |
Abhishek Gupta is an assistant professor in computer science and engineering at the Paul G. Allen School at the University of Washington. I lead the Washington Embodied Intelligence and Robotics Development (WEIRD) lab. Previously, he was a post-doctoral scholar at MIT, collaborating with Russ Tedrake and Pulkit Agarwal. He has worked on deployment of RL agents in human-centric environments with the focus on human-in-the-loop RL for targeted exploration and robustness, generalization and fast adaptation for robotic control policies.
|
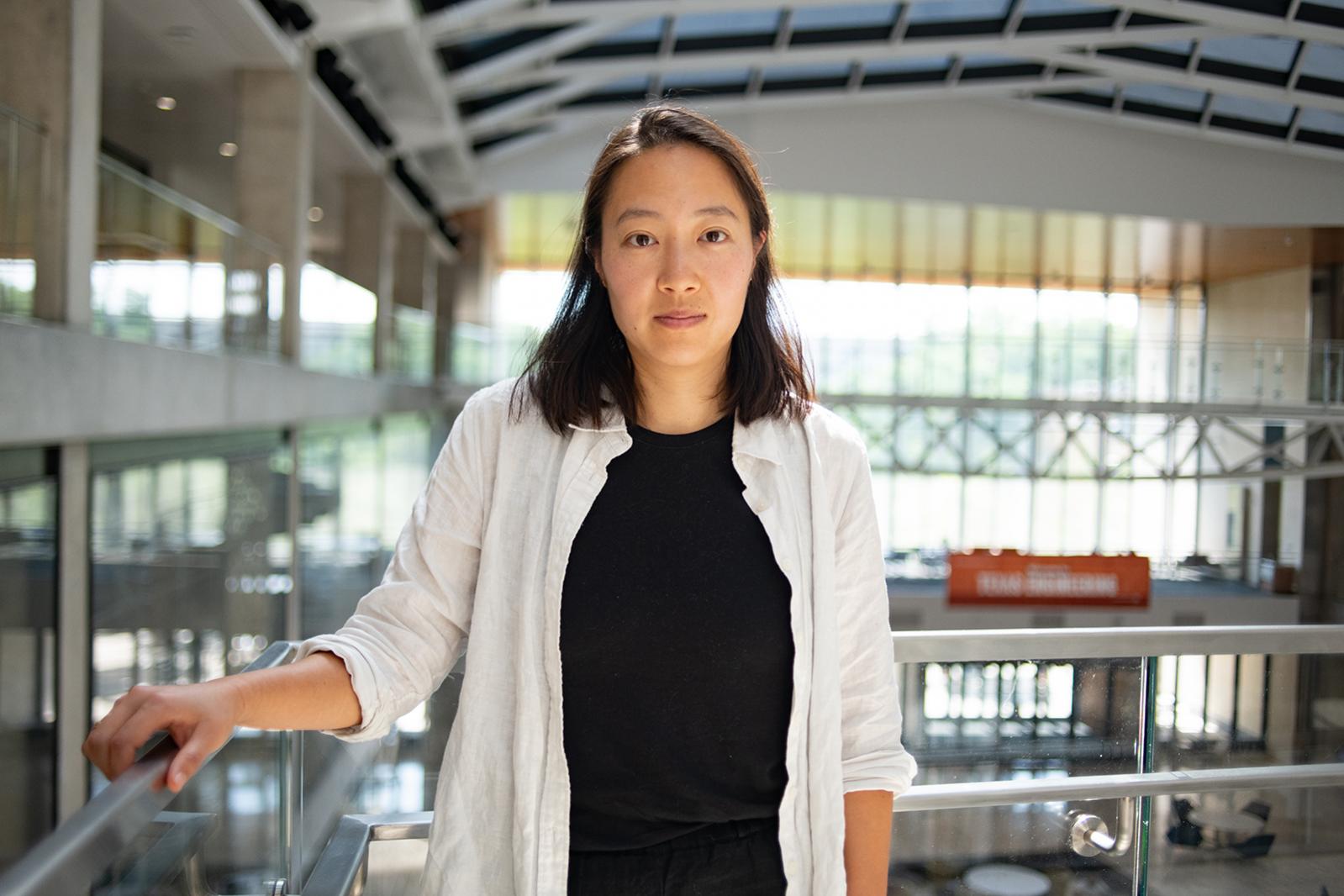 |
Amy Zhang is an assistant professor and Texas Instruments/Kilby Fellow in the Department of Electrical and Computer Engineering at UT Austin and an affiliate member of the Texas Robotics Consortium. Her work focuses on improving sample efficiency and generalization of reinforcement learning algorithms with a focus on representation learning. She completed her PhD in computer science at McGill University and Mila – Quebec Artificial Intelligence Institute, where she was advised by Joelle Pineau and Doina Precup.
|
 |
Ida Momennejad is a Senior Researcher in Reinforcement Learning at Microsoft Research. Her research investigates the intersection of reinforcement learning and human cognition & behavior, especially in determining how learning interacts with memory, exploration, and planning. Her work has explored human-like behavior and evaluation for model-based RL and language models. She received her PhD at Humboldt University in Berlin, Germany where she did research at the Bernstein Center for Computational Neuroscience.
|
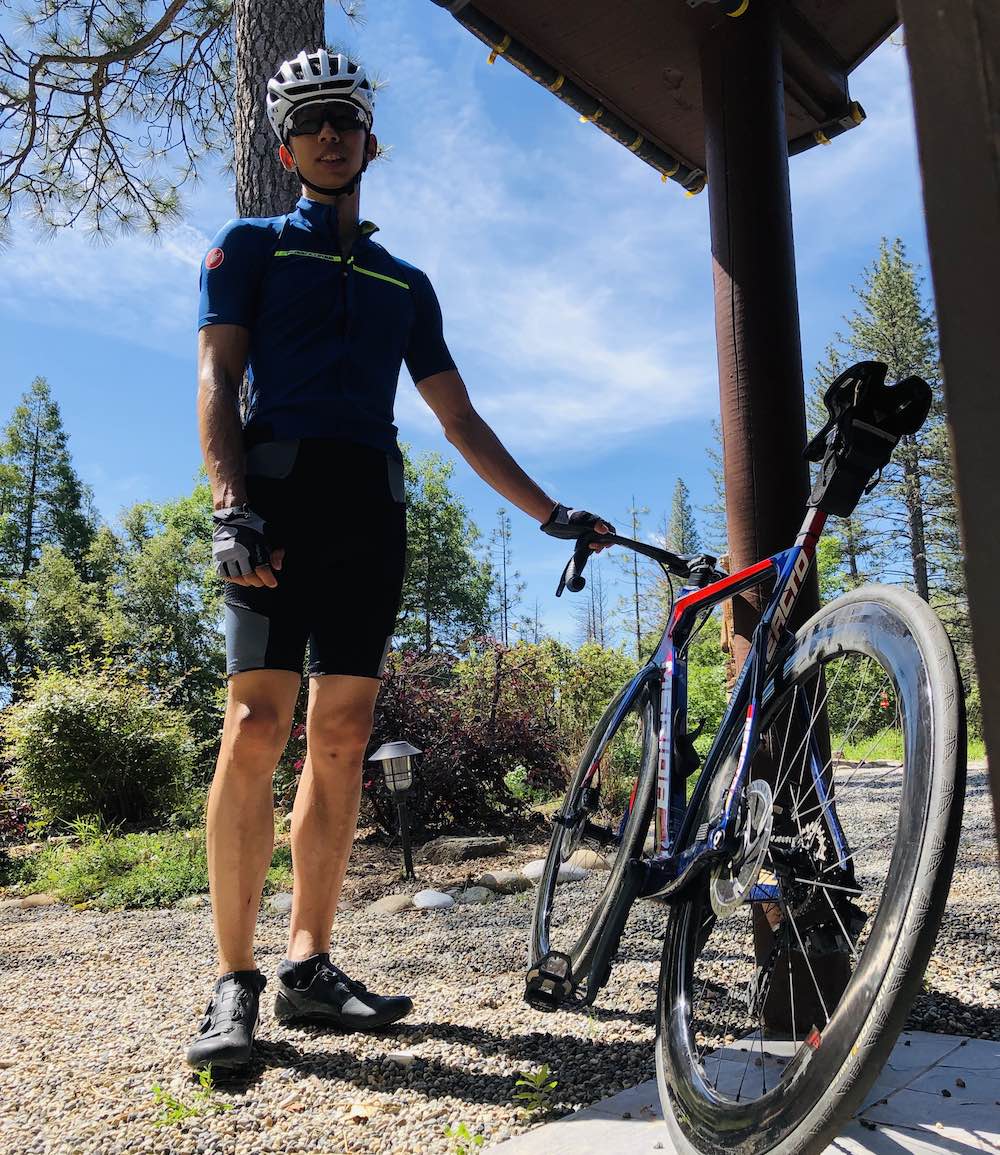 |
Fei Xia is a Senior Research Scientist at Google DeepMind where he works on the Robotics team. He received his PhD degree from the Department of Electrical Engineering, Stanford University. He was co-advised by Silvio Savarese in SVL and Leo Guibas. His mission is to build intelligent embodied agents that can interact with complex and unstructured real-world environments, with applications to home robotics.
|