Workshop on Reinforcement Learning Beyond Rewards
Reinforcement Learning Conference (RLC) 2024
August 9, 2024
@RLBRew_2024 · #RLBRew_2024
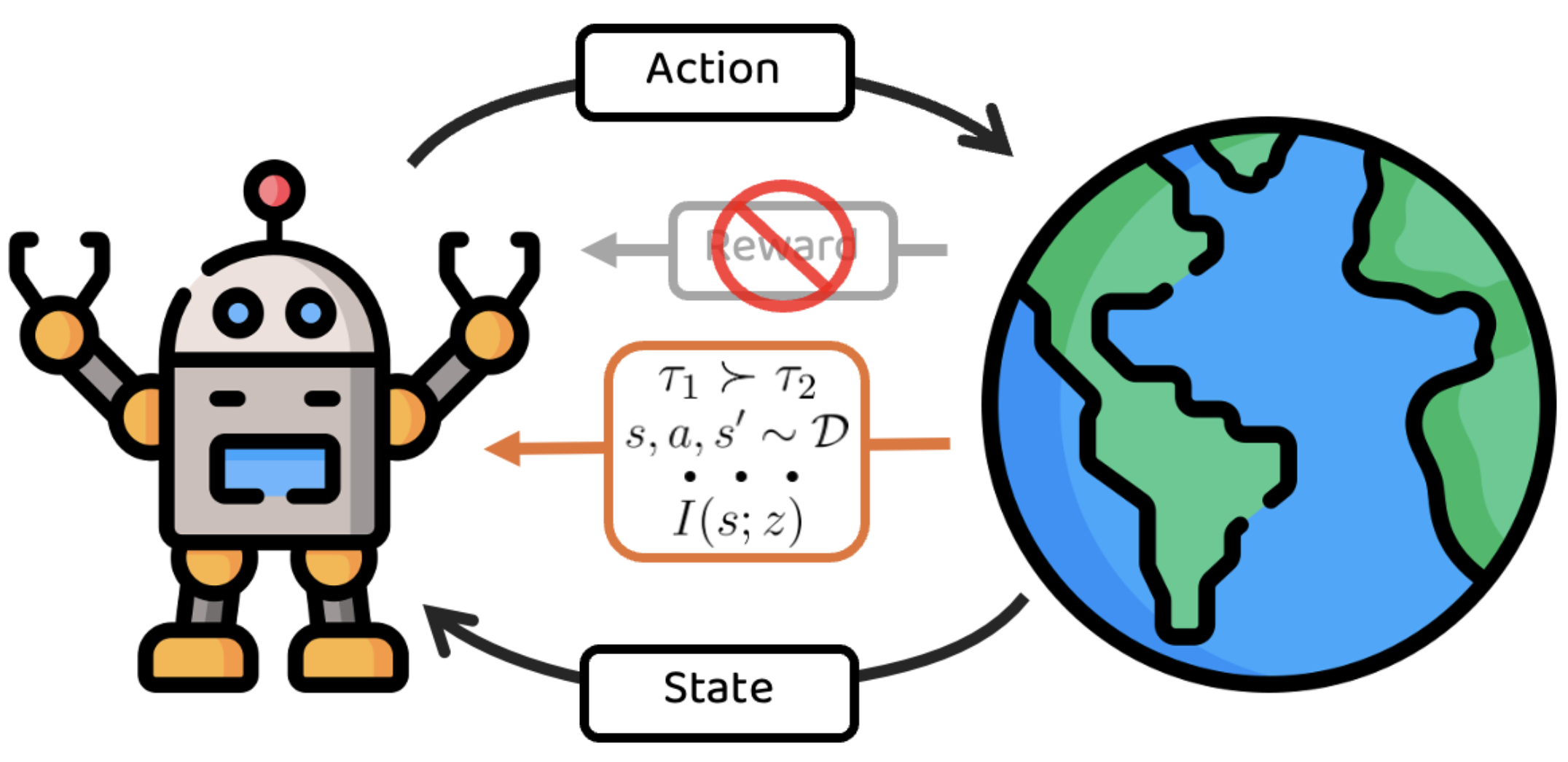
Reinforcement learning has been widely successful in solving particular tasks defined with a reward function - from superhuman Go playing to magnetic confinement for plasma control. On the other hand, creating a generalist RL agent poses the unresolved question of what agent can learn not just from reward-defined environments, but from the often substantial quantity of reward-free interactions with the environment. This question has been explored recently and has taken diverse forms—learning representations that are action-free, causal, predictive, and contrastive; learning from large-scale action-free datasets; learning exploration using intrinsic reward and skill discovery; learning policies that are arbitrary goal-reaching, language-conditioned, policies optimal for distribution of reward function, or even optimal for all reward functions; learning intent from datasets using a variety of learning signals like preferences, rankings, expert, and human cues; learning imitative foundational action models. The RLBrew workshop focuses on this setting of reward-free RL. Considering the wide variety of possibilities for RL beyond rewards, we aim to bring a set of diverse opinions to the table to spark discussion about the right questions and novel tools to introduce new capabilities for RL agents in the reward-free setting.
Organizers
To contact the organizers, please send an email to rlbrew.workshop@gmail.com or @ us on X/Twitter at @RLBRew_2024